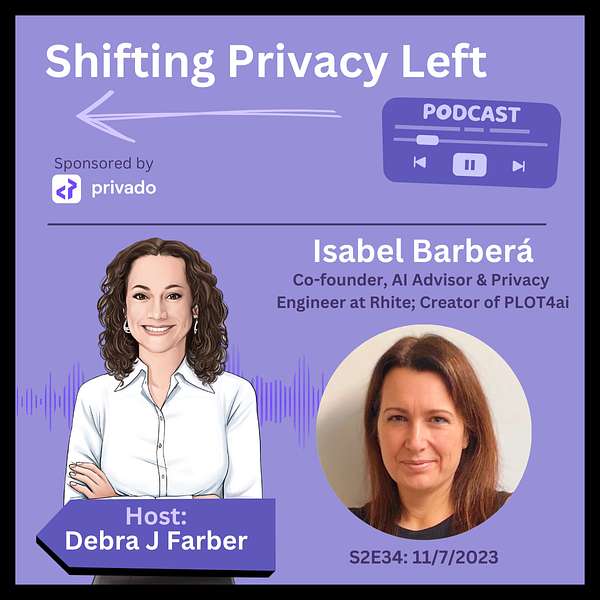
The Shifting Privacy Left Podcast
Shifting Privacy Left features lively discussions on the need for organizations to embed privacy by design into the UX/UI, architecture, engineering / DevOps and the overall product development processes BEFORE code or products are ever shipped. Each Tuesday, we publish a new episode that features interviews with privacy engineers, technologists, researchers, ethicists, innovators, market makers, and industry thought leaders. We dive deeply into this subject and unpack the exciting elements of emerging technologies and tech stacks that are driving privacy innovation; strategies and tactics that win trust; privacy pitfalls to avoid; privacy tech issues ripped from the headlines; and other juicy topics of interest.
The Shifting Privacy Left Podcast
S2E34: "Embedding Privacy by Design & Threat Modeling for AI" with Isabel Barberá (Rhite & PLOT4ai)
This week’s guest is Isabel Barberá, Co-founder, AI Advisor, and Privacy Engineer at Rhite , a consulting firm specializing in responsible and trustworthy AI and privacy engineering, and creator of The Privacy Library Of Threats 4 Artificial Intelligence Framework and card game. In our conversation, we discuss: Isabel’s work with privacy-by-design, privacy engineering, privacy threat modeling, and building trustworthy AI; and info about Rhite’s forthcoming Self-Assessment Open-Source framework for AI maturity, SARAI®. As we wrap up the episode, Isabel shares details about PLOT4ai, her AI threat modeling framework and card game created based on a library of threats for artificial intelligence.
Topics Covered:
- How Isabel became interested in privacy engineering, data protection, privacy by design, threat modeling, and trustworthy AI
- How companies are thinking (or not) about incorporating privacy-by-design strategies & tactics and privacy engineering approaches within their orgs today
- What steps can be taken so companies start investing in privacy engineering approaches; and whether AI has become a driver for such approaches.
- Background on Isabel’s company, Rhite, and its mission to build responsible solutions for society and its individuals using a technical mindset.
- What “Responsible & Trustworthy AI” means to Isabel
- The 5 core values that make up the acronym, R-H-I-T-E, and why they’re important for designing and building products & services.
- Isabel's advice for organizations as they approach AI risk assessments, analysis, & remediation
- The steps orgs can take in order to build responsible AI products & services
- What Isabel hopes to accomplish through Rhite's new framework: SARAI® (for AI maturity), an open source AI Self-Assessment Tool and Framework, and an extension the Privacy Library Of Threats 4 Artificial Intelligence (PLOT4ai) Framework (i.e., a library of AI risks)
- What motivated Isabel to focus on threat modeling for privacy
- How PLOT4ai builds on LINDDUN (which focuses on software development) and extends threat modeling to the AI lifecycle stages: Design, Input, Modeling, & Output
- How Isabel’s experience with the LINDDUN Go card game inspired her to develop of a PLOT4ai card game to make it more accessible to teams.
- Isabel calls for collaborators to contribute to the PLOT4ai open source database of AI threats as the community grows.
Resources Mentioned:
- Privacy Library Of Threats 4 Artificial Intelligence (PLOT4ai)
- PLOT4ai's Github Threat Repository
- "Threat Modeling Generative AI Systems with PLOT4ai”
- Self-Assessment for Responsible AI (SARAI®)
- LINDDUN Privacy Threat Model Framework
- "S2E19: Privacy Threat Modeling - Mitigating Privacy Threats in Software with Kim Wuyts (KU Leuven)”
- "Data Privacy: a runbook for engineers"
Guest Info:
Copyright © 2022 - 2024 Principled LLC. All rights reserved.

The privacy engineering profession - what I would like to say is that keep there; keep growing; but, also don't lose the view of the. . . it's not only technology. So, I'd like to advocate, for eventually, the the main goal is to really protect us all, whatever could be out there. So, we do our best with technology; but, eventually it's not only about the technologies. It's an effort for the people.
Debra Farber:Hello, I am Debra J. Farber. Welcome to The Shifting Privacy Left Podcast, where we talk about embedding privacy by design and default into the engineering function to prevent privacy harms to humans, and to prevent dystopia. Each week we'll bring you unique discussions with global privacy technologists and innovators working at the bleeding edge of privacy research and emerging technologies, standards, business models, and ecosystems. Welcome, everyone to Shifting Privacy Left. I'm your host and resident privacy guru, Debra J. Farber. Today, I'm delighted to welcome my next guest, Isabel Barbara, Co-Founder, AI Advisor, and Privacy Engineer at Rhite. That's R-h-i-t-e, a consulting firm specializing in responsible and trustworthy AI and privacy engineering. Isabel is a longtime leader in the field of privacy engineering. She is the industry chair for the International Workshop of Privacy Engineering, otherwise known as IWPE, Member of the ENISA, ad hoc Working Group on Data Protection Engineering, a National Expert at the Dutch AI Standardization Group that contributes to ISO standards, a Volunteer Member of the NIST Generative AI Public Working Group; and earlier this year, she was named "The Responsible AI Leader in the Netherlands" by Women in AI. So, in addition to advising private sector and public sector clients, Isabel also works with colleagues to conduct research on AI risk assessment tools and frameworks, and AI security threats and bias detection methodologies. And, they're creating an open source AI self-assessment tool and framework. Today, we're going to discuss her work with the privacy by design, privacy engineering, privacy threat modeling and trustworthy AI space. Welcome, Isabel.
Isabel Barberá:Thank you, Debra. I'm really happy to be here. That's a very long introduction.
Debra Farber:Well, you have so many accomplishments in your background, and I want to make sure everybody knows about them.
Isabel Barberá:Thank you.
Debra Farber:Sure. Why don't we kick it off with your origin story? How did you become interested in privacy, engineering, data protection, privacy by design, threat modeling, and trustworthy AI?
Isabel Barberá:So many things.
Debra Farber:You know it's gonna be hard to thread all that together, but I have confidence.
Isabel Barberá:Yeah. So, it was really long ago; I'm not so young anymore. Just funny, now, we are in the area of generative AI, and I studied, like 20 years ago, computational linguistics. Really funny now that other skills coming back. I've been working in the technical field really, really long; or in software development, architecture, and security. So, that, I was really curious. I always liked it, but I think the part of privacy is something that came to me because I have a really strong sense of what is right or what is wrong; and, I kind of remember the times when you're doing data migrations, and I was thinking "Can we really do this? Is it really. .. it's another country. Can really do those things?" It's legal. So, I was really busy with all those things. So yeah, that's basically how I started really long ago. I've been working really long for IBM, like more than 10 years. And then, after a while, I decided to go by myself, and really focus only on privacy and security. And yeah, AI at that time was mostly big data and some machine learning - something that I've be touching all my years, especially working for IBM, and then later in my career also. So, that when everything started for me.
Debra Farber:Fascinating. I think I share in your passion for 'advocating for what's right,' versus what's wrong. Deep, moral things propelling me forward to respect others and to tell other people to respect other people, as well. So, I think we share that.
Isabel Barberá:I think it's also really, really kind of a foundation in the field of privacy engineering; we tend to forget about that. I personally don't see it only as a technical solution of things, but really be sure that what is our responsible solution of how to implement these solutions.
Debra Farber:Yeah, absolutely. I don't think all privacy engineers do or have to have this sense of moral justice and right and wrong. It's interesting - just different motivators for different people. I know plenty of people, like Nishant Bhajaria, who wrote the book, the Runbook for Privacy Engineering, who saw privacy as an engineering problem in his organization, and he really gets a lot of value out of teaching others how to solve the problem of privacy in their orgs, but doesn't necessarily have that same motivating factor of we need to move forward the field because of ethics and justice. And not to say that he doesn't have ethics or sense of justice. It's just talking about different motivators of. . .
Isabel Barberá:Yeah, I think we just need each other. I work often in engineering teams, and we need all type of profiles. But eventually, I think the goal is basically the same: to protect dignity, to protect privacy. So, the technical solution is something you need; and that's why we need all these technical profiles. But, I want to believe that eventually, it's all towards the same goal.
Debra Farber:Yes. So tell me, how do you see companies thinking about incorporating privacy-by-design strategies and tactics and privacy engineering approaches within organizations today? And I guess, are they doing what they should be doing? Or how are they thinking about it; and then how should they be thinking about it?
Isabel Barberá:Not sure you should have asked me that question because, at least in Europe, I don't really see. . .I don't see a lot of attention to privacy engineering. It's true that I see, especially the last few years, more attention to try to build solutions in a better way, but not so. . .still we don't go so technical. I like the fact that privacy enhancing technologies is not something. ..I mean, it's really growing; but still, organizations are really careful. One of the reasons is some of the of the solutions - there is not really.. .how do you say this. . .you don't really see always that they work 100%. So, they don't offer you their 100% warranty. So, some of them are still research models.
Debra Farber:Some of them are still research in the lab, and not necessarily deployable at scale within companies. Is that what I'm hearing?
Isabel Barberá:Yes. That's one of the reasons. Another, there's a lot of implementation costs. I mean, there's unfortunately no check, like one-size-fits-all solution. That's where I see, in practice, our organizations want. So, you're right there, and you're telling me that you just have to click the button, and then you have a solution here. But, it doesn't work like that; you need to really do an implementation process, which sometimes takes quite sometime, some months. But, it also means you need extra costs, extra resources that need to be available; and, often it is not the top of the mind for organizations. You also see a lot of focus in compliance. As long as there's kind of a DPO with a legal background that can more or less sort out things quick, that's fine for the time being, until then you can really focus on the type of solutions. This is not all organizations. I mean, especially in the financial sector, in the health sector, that is really more open towards implementing privacy engineering, but not so much as I would like to see.
Debra Farber:Right. There's so much opportunity for educating.
Isabel Barberá:That's really true.
Debra Farber:It's great to hear your feedback, especially in the European market; because, of course, that's the same here in the U.S. too. Although there are some of the BigTech companies are doing some more investment in processes and engineering, which I know is more stateside. So, we hear a lot more about it; I hear more about it focusing on the space. So, what's it going to take to educate companies adequately so that they start investing in privacy engineering approaches? Do you think AI is a driver?
Isabel Barberá:I think maybe one of the things is maybe more collaboration between the academic world and industry is necessary. More priorities that are really a mix, like the academic world trying to solve issues from industry; but not only from really big companies, but also SMEs, smaller companies- probably for that we need some financial resources within the European level, that data sharing strategy. Here we see it now with all these revelations that have come. That is really the drive - to share data and to get value out of it, but still protecting individuals. So, the only way to really do that is implementing right engineering, privacy-by-design. There's definitely a lack of resources, but also a lack of understanding that it's not just a technical solution and that's all; you That's what I also see, now often, that legal teams are a really need to still look at the whole picture. bit afraid, like, "Well, you come with a technical solution, but there's still other things to cover." I keep saying that, of course that has to keep happening. You still have to look at the whole picture to see what can go wrong here. What what type of risks do you need to mitigate? What type of issues? The whole story is not one, like I said before, solution that fits all the problems and that's all; we still need to see that it's not easy. For that, I think that we need more collaboration, that beautiful things are happening in the academic world. In industry, we don't have chances to do this type of implementations; at least, not possible organizations. So, more collaboration, more financation, more resources, are absolutely necessary. And more awareness of what really is privacy engineering, or what could be eventually.
Debra Farber:Yeah, I for one would love to see more for-profit organizations creating collaborations - whether it's standing up a nonprofit, or putting money towards it, or some sort of open source cross dialogue, even with competitors to talk about some of these things and privacy, the way that we've seen in security. Right now, I feel like no one wants to admit where they are in their process because they don't want to be critiqued by others. So, you know, organizations... unless it's some major win, come to market and use as a marketing tool, they're keeping their strategies and tactics close at hand; and unfortunately, that means others can't learn from mistakes they've made or wins that they've had. So, I definitely think we need something like that - a big advocate of it. I'd love to plug into an organization like that, personally, if it existed.
Isabel Barberá:Yeah, I agree with you.
Debra Farber:So, let's talk a little bit about Rhite and your work at that org focusing on privacy-by-design and responsible and trustworthy AI. First, can you tell us a little bit about the company and its mission? (and I know that it's beyond just responsible and trustworthy AI and privacy by design). If you could give us a some background on the org, it would be great.
Isabel Barberá:Yeah, I found it Rhite not too long ago, one year ago in the summer of 2022. It's something I had in my mind already for so long, like my dream really to focus on really the privacy engineering side. The way I look at privacy engineering - of course, because I'm in the academic world, and there are a lot of focus for the area: the technical privacy engineering, data protection engineer, but but I wanted really to stem that to what I consider is more of a responsible way of building solutions and data solutions. So, not only with the attention on the data protection engineering side, but really questioning yourself, "What can go wrong here?" Is it really meaningful to build this? What the impact can really cause for the individuals and societies - really go beyond that. Something that I've experienced myself often, working as a privacy and security engineering teams, where we were really focused on the technical side, and suddenly had to step back and think about where are they going? What are we building here? Is this really something we think people will react badly? So, what type of impact we could really cause over the short or the long term? So, those are things you need to. ..I'm sure those are things you need to really consider when you're building solutions; and not only building, before you're just going to use any data solution. That is why I started to co-found Rhite. That's basically the mission: how to really build responsible solutions with society and individuals in mind, with a technical mindset.
Debra Farber:I really love that because it's just thinking socio-technical. It's not just,"I'm building a product and here are the requirements for the product." But, it's also like, thinking about impacts beyond the product, thinking about the customer, thinking about society, thinking about, like you said, what's responsible and trustworthy? And so, basically, what I want to know from you, what does 'responsible and trustworthy AI' mean?
Isabel Barberá:Yeah, that's also a really good question because who knows that?
Debra Farber:What does it mean to you at least?
Isabel Barberá:It's all about.. . at least, like before a few years ago, it was all about'Responsible AI,' and especially I think, in the U.S., it was mainly 'Responsible AI.' Then in Europe came the 'Trustworthy AI,' also went there, the'Ethical Guidelines' that were published. And then, we have this confusion, like, "But, wait a second, then is it'Responsible AI,' or is it'Trustworthy AI'?" I mean, I also saw the movement in the NIST website, where it's'Responsible and Trustworthy AI.' So, now they use the two
terms and nobody really knows:what is really the difference? You hear a lot of different answers to that question. I like to look at it like at some point, I mean, responsible, you know, you're doing or you should be doing the right thing, maybe more related to, at some point, compliance; that's how I also see often more use outside. And, we trustworthy, you really want to transmit that sense, to the user or the end user, that you can trust what I'm doing. So, I'm more transparent, not just responsible. Because 'we're responsible' can also mean"Okay, I'm compliant," but that doesn't mean I want to be responsible. Sometimes you can do things by law and it's okay, but that doesn't mean you're being responsible and thinking of the impact. Still, there's other versions of that -'Trustworthy,' in terms of trust, that we use a lot in security, too. So, I will keep it just like that, and let the audience to decide by themselves. For myself, I don't really have an answer to that. I tend to use both. Sometimes it's more of a marketing thing. So, you have to position yourself as responsible AI. I more like'Responsible Data Solutions.' Like I said, let's just keep it together.
Debra Farber:Yeah. Yeah, it makes sense. The only other word I don't see there that they all seem to be used interchangeably is 'Ethical.' Right? So'Responsible, Ethical, and Trustworthy AI.'
Isabel Barberá:Yeah, I find'ethical' a bit tricky because, I would say more like. . .
Debra Farber:It's a judgement.
Isabel Barberá:Yeah, we use a lot the term, but I think in engineering teams, it's too abstract, so we want to really translate that into requirements. And eventually, I mean, you can translate it into law. I mean, we have fundamental rights. There's ways to translate that, but when it comes to just ethics, it's so. ..I like to get more in the
dilemmas thinking:"Who are really your stakeholders?" Who are you going to create impact with what you are doing and not realizing that there are some dilemmas that you could have there, and that you need to give a solution, to try to find a balance there. But, what's the answer to ethical issues? It's probably, for engineers, becomes too abstract that discussion.
Debra Farber:Right. It kind of does. So, I understand from your website that Rhite is an acronym for 5 core values. So, R-H-I-T-E. Can you share with us what they are, and why they're important for designing and building products and services?
Isabel Barberá:Sure. So, the'R' is for 'Responsible' - I think we were already talking about that. Then, we have the'H' for Human. So, of course, always the human-centered designs with the idea of, of course not always, but when you are really building something that could have impact on society or individuals, we consider that a core value. The'I' is for 'Ingenious,' meaning that you build things when thinking about society in a responsible way; that doesn't mean that you are not using clever, intelligent, and a good way to really build things. So, technology and good intentions; it's also intelligent; and, it's also necessary. I think, especially in the field of responsible design, privacy engineering - is there something really in creativities find value. Then, the 'T' is for'Transparency,' which is really important, also trust. Of course, it already implies that if you're responsible, eventually you are transparent about what you are doing. I also strongly support open source software, for instance. The last one is 'E' for 'Empathy,' one that I find extremely important and that I don't see so often. If you cannot place yourself on the place of the other, it's difficult that you can really build solutions that are protecting others or have really an impact on others. Asking yourself, "What can go on and affect others?" you need to place yourself in that position to try to feel what others would feel. Maybe using your technology, you go through the user journey, for instance.
Debra Farber:That's awesome. So, besides being values for your company, do you, I guess, map activities and deliverables to these values, so that they're shown to the clients? Or, are they more internal values for employees
Isabel Barberá:You just gave me a really good idea. At this moment, they we're just internal values. Of course, they're also mapped in the things we do, like the assessments we do, and the tooling and the research that we are doing. So, it's one way or the other, it's also up there. But no, we're say they're mostly internal values, and I think, yeah, you just gave me a really good idea.
Debra Farber:There you go. Yeah, because I'm just thinking, how do you impart those values on to the some of this systems and the processes that you're helping to develop for the clients? How do you embed that into what they're building? There's an idea for the future.
Isabel Barberá:That's great. Yeah. And eventually, it also depends so much on the use case and the organization where you are. So, you try to adapt to the culture of the organization, I always underscore the importance of being transparent. So, those are most important values. Also as organization, decide which type of clients you want to work with. Some things are not really like that. And probably, you don't want to be there. So, if that's not only the design of tools, but also the way that we are ourselves.
Debra Farber:That makes sense. That also is a good reminder that internal value statements like what you've created really does help provide the constraints for who you want to take on as a client. I love that. I think that's really helpful. So, many companies right now are deciding whether to bring different types of AI into their organizations in many different ways. But, they are not sure which uses and deployments will bring privacy, security and safety risks into their organizations. That said, how are companies approaching risk assessments, analysis and remediation of AI harms today? And, I know a lot of them might be polarized and not necessarily doing anything. So, what I mean"today" - and it could even be advice you're giving today, as opposed to what they were already doing before you gave them advice. Like, what are some of the approaches?
Isabel Barberá:Yeah, and I see nowadays, especially since generative AI - probably say more ChatGPT has become really booming. So, organizations are also wondering theirselves, "Can I use this? Is it dangerous?" Especially now, there are questions regarding copyright issues and the data breaches that popped up. Can I really use the tool? What happens with with my data? Is it's going to be used by the organization to train their models? So, what's going to happen here? There's also a lack of the knowledge and expertise. Not all these applications, if they're not open sourced, and it's also difficult to give the right answer. So, you need to be careful with what you do. How the organizations are doing this, I see often, the DPOs or Privacy Officers are the one asked (or maybe CISOs and different security departments. I will say, to do threat modeling, because honestly, it's not something I see often happening in practice, but at least to do: a risk assessment and look at the profile of the organization; maybe a threat landscape, what type of information you're gonna share, what type of impact that we have, maybe to post ourselves a custom model or wherever, depending on the circumstances, and then take decisions based on that. I see a lot of confusion at this moment. What happens also is that even organizations would like to ask third-parties for more information, they don't know what to ask. Even if they ask, they still don't know how to analyze the information they get from them. So, there's a bit of this moment, like, "Who should really help us here?" In practice is where I mentioned before, especially Legal teams that write some guidance for teams - "Be careful with this. Be careful with sharing data here or there - basically that's how we are surviving nowadays.
Debra Farber:That makes sense. It sounds like there's confusion in the market, both for those who are selling applications built on LLMs. Or if they're just selling access to LLM to an organization, there's all these questions of like, "You know, I want to do the right thing. I want to make sure it's safe, but don't really know what to ask." And then, those who are selling it don't know how to necessarily and confidently be able to explain how their clients can make it safe.
Isabel Barberá:They're learning themselves. I think probably OpenAI would be one of the ones that, you can say, the last one is is learning more for all things that are happening and all the questions they get. And then they realize, "Oh, we have to implement these. We have to do this." I think that's what's happening nowadays in the AI world. I mean, all these companies are learning themselves. Yeah, suddenly, there's compliance, there's rules, data privacy (that some of them I also forgot was there). Things just happened really, really fast. I just say,"Yeah, it's a pity, because at least data protection law is something we already have there." I see it for me, there's no difference, no matter if you're building AI, you're building any other application. But especially, in the world of AI among the startups, there's really a lack of knowledge or awareness that they have to do things probably in a different way, or at least have really careful assessments and think themselves, "What should do to comply? Is my product even going to be eventually. . . am I going to be able to launch into the market is especially laser when we have the EU AI Act enforced. And yeah, that's what I see now in practice. I see, especially that - the lack of awareness, lack of understanding. . .is really the focus on the technology, the solutions that are really cool, all of them; but, without really paying attention to other things that are important. It's a learning process. They will need to learn, and I just hope in the meantime, not too many issues and incidents happen.
Debra Farber:Yeah, it's definitely growing up. I think.. .I've never seen a technology come to market so quickly, where everyone's trying to run and figure out how to how to use it in a way that, not only is it making it safe, but that they can avoid regulatory problems in the future.
Isabel Barberá:And so accessible, also, because there's a lot of open source AI available, too.
Debra Farber:That's a good point. It's accessible no matter what your budget is; you can find access and capabilities to leverage it. Yeah. That said, what activities are required for organizations to feel confident that they have built AI responsibly. How do you guide organizations through this? Or, what are the steps they need to take to be able to build AI responsibly.
Isabel Barberá:Of course, I'm an advocate of threat modeling. So, please try to find out what could go wrong with your ID. That is something that when I mentor startups, I try to. . Talk to me about that, the data governance aspect, then..especially, I do as I should with my PLOT4ai Threat Modeling Game, and they come in merely to. . .it's like an eye opener like, "Oh, suddenly I see this. So we should correct this. Maybe we should do this. Maybe we should do that." Of course, taking care of your own governance and your data quality, for instance, those are things that I see in practice. They don't happen. Especially in startups, it'ss something that you really don't see. I mean, they don't even think about that. Then, you have the Data Scientist, the Machine Learning Engineers there. Everybody's doing their stuff, sharing the models. I mean, there's not really an understanding that the things also need to be protected. Who is responsible for what? Who has access to, for instance, certain data sets? Who could, for instance, have access to certain models to change parameters? I mean, all the things should be.. .if you have an audit trail, you have control. Of course, that helps to avoid issues. A thing I also see in practice, a lack of understanding of what can go wrong with bias, for instance. I mean, we all talk about bias and discrimination; but later, the teams don't know very well how to test that for that. There's a lot of tools there; but of course, you need to know which tool for your specific use case. You need to know how to interpret the results of that. It's also really a lack of understanding. Of course, that is when you can, eventually, you can have issues. You need to also monitor what you are doing, so that is part of the governances. So, you keep monitoring your pipelines; you keep monitoring what you are doing; you can react to incidents; you are able to see that. The type of responsibility building your process, where you can supervise what is happening and you can react when something is wrong, is something that I don't see often in practice. And eventually. . .it also depends what you are building. Eventually, you have some risk. But I see that, not only in AI; but, in any type of software, we try to peel things in a structured way - understanding who has access here and all these controls you build around your whole architecture. So, why not with AI? We tend to forget that it's not so different. It's a technology, so it has to grow in maturity, in that sense.
Debra Farber:I suspect that it will grow and there'll be frameworks and such that help with this at a global, or at least even a national level and global, maybe an ISO standard; but, these things take time. So, until we actually have official standards for these things or frameworks from government and public sector-private sector collaborations, right now, we just got to start dealing with best practices to achieve the goals and like learning from one another, and all that. I know that you, together with some colleagues at Rhite, are working on creating an open source AI Self-Assessment Tool and Framework called SARAI. Is that it?
Isabel Barberá:Yeah, it's SARAI, which is the name of a girl. What it means is Self-Assessment Tool for Responsible AI.
Debra Farber:There you go. And, you're going to be publishing next year. Cool. So, tell us more about this effort. What do you hope it accomplishes?
Isabel Barberá:SARAI is kind of an extension PLOT4ai, and we didn't talk about that.
Debra Farber:We'll talk about that next. Yeah.
Isabel Barberá:Let's go first to the future; it's alsogood. But what PLOT4ai is - I need to mention this for everybody to make sense of what SARAI is. PLOT4ai is a library of AI risks. It contains more than 80 different risks, and they're categorized in 8 different categories. The thing is, what I thought already more than one year ago, what I see, there's a lot of need for understanding how to really promote responsible AI at a more global level. So, not only at organizational level, but also at your product level, your model level. I will say that a lot of the information is already out there, and a lot of the things are not so different from what we usually do in practice, especially if your organization is a bit more mature. But there's a lot of"Blah, blah, blah." Everybody talking about responsibly AI and ethics, and this and that, and other instructions - really important things, like the protection of fundamental rights. But, when it comes to engineering teams to organizations, there's a lot of confusion. "I really need to do this. I have to do this." It's really something so so different, but there's not really guidance about that. What I started to make was based on the risk, PLOT4ai, I created controls. There's more than 100 controls. And for control, variable control, you have mitigations, recommendation to mitigate that specific risk(also coming from PLOT4ai and some of them), and also recommendation to collect evidence. So, basically, the system tells you "By having this type of evidence, you could be basically implementing that control." So, that will really help you to implement this control and then eventually prevent this risk. There's a complex data model behind SARAI because it basically integrates all when it comes into our hands. So, what is available in terms of standardization to ethical frameworks to always there, we have tried to make a division and a categorization of the controls that makes sense to eventually try to contain 6 blocks - every block covering different principles and different controls. So SARAI will help you as organization to understand what type of protocols and processes you could implement to really comply with controls, and will help you to avoid certain risks. That, like I mentioned before, at the organizational level, product level, there's like two different sections: 1) if you are building the tool or if you are purchasing it the tool, and 2) eventually, at model level. It's quite complex, like I said, but eventually, it tends to be really easy to understand and that can help everybody to set the first steps towards responsible AI. As I mentioned it before, I'm really fond of open source, and when I have things on my mind, I like, with help of my college, work in often in my free time to really deliver things that we think, "This can really help community." Eventually, the goal is simply to protect individuals, to avoid risks, and to make things easier for everybody; and get out of this Wild West mentality, especially in a world that I see, where everybody's trying to sell solutions and new things, sometimes are necessary, but I think first you need to have an understanding of what you need, and all the things that are already there as open source. For instance, like the model cards, data sheets. I mean, all those things are there. If you facilitate a way to have those things, to integrate with Jira, I mean, there's things to really make life much easier for organizations (and not only startups but for everybody). So, that's the essence of SARAI.
Debra Farber:Thank you. So what I'm hearing for SARAI is that, it's not just a self assessment tool and framework, but it also helps you - based on that assessment - with your next steps and like what tools to use and how to integrate with things to achieve goals.
Isabel Barberá:Yeah, it's much more in fact, much more than a self-assessment tool. It will also provide you with a maturity level assessment. So, it helps you to grow as organization, because it's under the assumption that not all evidence needs to be provided. Like I said before, it is a complex data model there because all of this is also based on the type of tool that you want to build or the type of tool that you want to purchase. So, this kind of a risk analysis behind them, that is why, depending on that, you need to cover for certain controls and certain risks. Then, you can really grow in that central organization towards a more mature level. So yeah, the tool contains several things. What we plan to release beginning next year is the tool- not the technical tool, but just the information - so the model for people to use it. We're, on the side, also building a tool because it will be much easier to have these assessments and controls. That will take a bit longer, it won't be a beginning of the year, but.. .
Debra Farber:So, sometime next year, you'll publish the tool.
Isabel Barberá:Yes.
Debra Farber:But first, you'll publish the framework.
Isabel Barberá:I believe, especially in smaller organizations, I believe they will benefit from the beta version; but, I think everybody can benefit already from what we're going to publish. So why not?
Debra Farber:Yeah, that helps also just educating the industry. It just helps everybody. I love it. Thank you for all that work. Okay, so let's turn our attention to privacy threat modeling, what motivated you to focus on threat modeling for privacy? And I think you mentioned before - it is a subsection of privacy engineering; but, why is that a feature for your consulting firm?
Isabel Barberá:Identifying risk is kind of a foundation for whatever you want to build. That's how I see it. And it also, I think, probably I mentioned before, the foundation of what makes you really build responsible solutions - if you don't question yourself, "What can go wrong here?" and you make that step back, like I said before, and say, "Wait a second, what is the impact here?" beyond that, then it's really difficult to build possible solutions. Why Threat Modeling? I've been working in the field of risk management really long - I think, from the beginning of my career - and I always like to do it in teams and to brainstorm about, "Okay, let's think what can go wrong here?" I also have security background. So, if you use, for instance, the team was tried, then of course, is a different way of approaching it- more often from the threat actor position, the attacker, and you, most of the times, at least, that's how I mostly experienced in the sessions you need to people with more of that kind of ethical hacker or technical knowledge. But, when I do risk management from any other solution, that is not only looking at the security side, but just you need to create an impact assessment; and that is something I remember very well in working for IBM was like always the first step, we had to create an impact assessment. And for me, I know, for some colleague was like, "Why'd you just do alone your impact assessment?" and I never agree with that. So, I don't have all the knowledge. We need to sit together with some stakeholders. We need to look at what is the impact of this where we want to be? And that has been one way or the other since the beginning in my career. Like I said, it's always really a foundation of what you are going to do in your next step. To ask about privacy specifically, I've been working with Linddun.
Debra Farber:Tell us what LINDDUN is. We did one episode on it with Kim Wuyts. She's wonderful, and everyone should listen to that episode as well to learn about the LINDDUN Threat Model Framework. Tell us a little bit about what LINDDUN is just for the listeners who did not hear that episode.
Isabel Barberá:Yeah, LINDDUN is a privacy threat modeling framework, I will say more focused on the development of software, any type of software. So for me personally, it's probably not the best solution for artificial intelligence. In fact, that's the reason why I actually create PLOT4ai. And LINDDUN has also the section called LINDDUN Go, more kind of applied version methodology that provides us with a set of card decks. And that is really nice because also what inspires me to create PLOT4ai. So, with the cards, it really helps motivate the teams to thinking what can go wrong in the data flow diagram that you design beforehand. So, I thought it was really nice to work with; and then, I have, in that sense, experience with the things where I've worked, using LINDDUN. And that is what moved me eventually, like I said, to create PLOT4ai. In 2019, I was visting one of my clients, and I realized, "No, I have to extend LINDDUN. I'm missing out." I mean, I was aware of that I couldn't in the methodology itself, and that is eventually the reason for me to starting extending the game into what it became in 2022 - PLOT4ai.
Debra Farber:That's awesome. And I'm definitely gonna be asking you next about PLOT4ai. Sorry that I put it at the end. So, to set the
Isabel Barberá:Finally! scene, Isabel is talking about cards that are a card game based off a 3 year research project that you've done, and it's called PLOT4ai, which she'll talk about in a second, which is an open source privacy library of threats for AI, which contains more than 80 AI threats with recommended mitigations. And that's so cool! Right? I want to hear more about this because my audience of privacy engineers are certainly going to be excited about what you're putting together here. So, you gave us a little bit about your background and why why you felt you needed to create PLOT4ai because the LINDDUN threat model is more aimed at software development and doesn't really focus on the AI space. Anything more on the background that you want to talk about there, or what PLOT4ai stands for, before I ask you a few other questions? Yes, it stands for 'Privacy Library of Threats for Artificial Intelligence'(PLOT4ai). I tried to make a game - a kind of acronym as the word 'PLOT,' which is often used in biostatistics in general. Something important maybe to mention about PLOT4ai is that when I was researching on threats related to AI, I realized I really had to go out of my comfort zone. What we are used to seeing in frameworks like LINDDUN, where we look really more pure at the privacy engineering issues. Of course, there's also compliance threats; but, with AI, it's so extensive. You have a lot of issues related to human rights; for instance, the possibility to cause harm in that sense. It goes beyond'safety.' Also, it has a lot of more technical, specific issues while advancing security, that is also. . .not all security, of course, because in general, it'ss basically the same ones with any other technology, but they're threats really specific for AI. That makes it. . . it becomes kind of a monster. So, when I was researching this, I realized to make it more accessible and to keep investigating this so it will become a way for me, as a consultant / advisor, to build my own library of threats for artificial intelligence - like I mentioned, kind of an extension of LINDDUN, to make it work for myself. Why it became really three years research, until eventually I published it. It's still keeps being developed. I mean, all what comes into my hands or guidance or a new standard - all is there. I updated PLOT4ai, and it's based on more than 200 sources of information. So, based on all that, is how I identified risks and I categorize those risks. I kept the essence of LINDDUN, so I use the similar colors for the cards and also maintained some of the names of the categories of LINDDUN - for instance,"Non-compliance" or"Unawareness" - that all that comes from LINDDUN, but eventually it has his own identity.
Debra Farber:Yeah. I definitely want to get my hands on this card game, so we'll talk about that afterwards. But, I want everyone to know - I'm sorry, I'll also put links to PLOT4ai and the game and the entire library - that link I'll put in the show notes so everyone can have access to it. And, the game costs, according to your website, 25 Euro. I want to give you my money and hand it over to you because this is really helpful - even just to have them on hand as I talk to other people. But, I also want to play the game; that sounds like fun. I also want to put out here that...I want to read what the categories are. You've created 8 different categories across 4 different aspects of AI model creation: Design, Input, Modeling, Output stages. In the Design stage, you'd be looking at Technique and Processes and Accessibility. In the Input stage, you'd be looking at Identifiability and Linkability, as well as Security. In the Modeling stage, you'll look at Safety and Unawareness - which I'm going to ask you about in a second, as to what that is. And then, the Output stage, you're going to look at Ethics and Human Rights issues, as well as Noncompliance - whether with laws or your own standards within your org, but just noncompliance issues. What is "Unawareness" in the Modeling stage?
Isabel Barberá:Yeah, unawareness is lack of information. So, it's basically all risk related when you are not giving the right information to individuals or data subjects with privacy. I need to make a small correction to all what you said, because you mention all the categories for for PLOT4ai. So it's true, 86 (at this moment) risks - or threats, sorry - are categorized in in these 8 categories, classifyied as these 8 categories. The 1st categorization in the phases of design, input, modeling, and output is just for threats. So, what you see - the game just gives you a suggestion in which phase of the development lifecycle of the AI it's more probable that that threat will arise. So, it's not the specific categories that appear and are specific from our development lifecycle. It's just that some specific threats could appear in all the phases of the development lifecycle or only two of them. So, there is more than the suggestion.
Debra Farber:I see, so these are the threat categories that would be presented at those stages.
Isabel Barberá:Exactly. So, with every card from PLOT4ai, it's an indication to which categories. For instance, a threat could be from a category, Accessibility. Then, you can see on the right side of the card that, for instance, appears more often during the design phase and the output phase. So, that helps you to - when you are doing your threat modeling - that you know if you're already in the phase with your data preparation, or you're already building your model, that that threat has been a suggestion, but probably you don't need to go through it again because it appears mostly in the design phase or during the output phase. So, you will need to do it again to use the card again during the output phase, once you have deployed your systems, for instance.
Debra Farber:Got it. Okay, thanks for that correction. So, I know that this is open source. How can other others contribute to PLOT4ai and what type of perspectives are you seeking?
Isabel Barberá:Yeah. Together with my partner, we built a website. Also, within a escape in GitHub. So everybody can collaborate in GitHub, send feedback, send new threats, send corrections because PLOT4ai, of course, is based on all the analysis of, like I mentioned before, a lot of sources of information, but also my own expertise, of course. I'm not here 'the guru;' I don't know everything. There are awesome people there outside who can really share the knowledge and make it community-driven, too. And the same goes for for the card game. You mentioned before about that 25 Euro - I really sell the game without profit. So, it's the same price I get from the from the printer. So anyway, in the amount of boxes that I bought, that's how I sell it. As always, the privacy engineering profession, what I would like to say is that keep there, keep growing; but also, don't lose the view of. . .it's not only technology that I like to advocate for. Eventually, the main goal is to really protect us all from whatever could be out there. We do our best with technology; but eventually, it's not only about the technologies. It's a mutual effort for the people. Regarding AI, yeah, just collaborate with PLOT4ai, which will help to create a more with the world. AI will really just make it fun. But yeah, I will be really grateful as the community grows.
Debra Farber:I agree. I think those are great words of wisdom- that there are humans behind the personal data. I think that's uniquely different from other areas, like security, or.. .I mean, obviously, you have to protect and secure people, but a lot of security is about securing systems and networks and things that have nothing to do with personal data. Right? So, privacy is contextual and all privacy has to do with...and data protection...has to do with people behind the technology. So, I think that's a great way to close today. Remember to think of the people and protect.. .
Isabel Barberá:Some beautiful words, and I think sharing is caring. So, let's work together to to make it better.
Debra Farber:Well, thank you, Isabel for joining us today on The shifting Privacy Left Podcast.
Isabel Barberá:Thank you for doing such a great job for advocating for the profession.
Debra Farber:Oh, you're very welcome. Thanks for joining us today. Until next Tuesday, everyone, when we'll be back with engaging content and another great guest, or guests. Thanks for joining us this week on Shifting Privacy Left. Make sure to visit our website shiftingprivacyleft.com where you can subscribe to updates so you'll never miss a show. While you're at it, if you found this episode valuable, go ahead and share it with a friend. And, if you're an engineer who cares passionately about privacy, check out Privado: the developer-friendly privacy platform and sponsor of the show. To learn more, go to privado.ai .Be sure to tune in next Tuesday for a new episode. Bye for now.